AI Drug Discovery

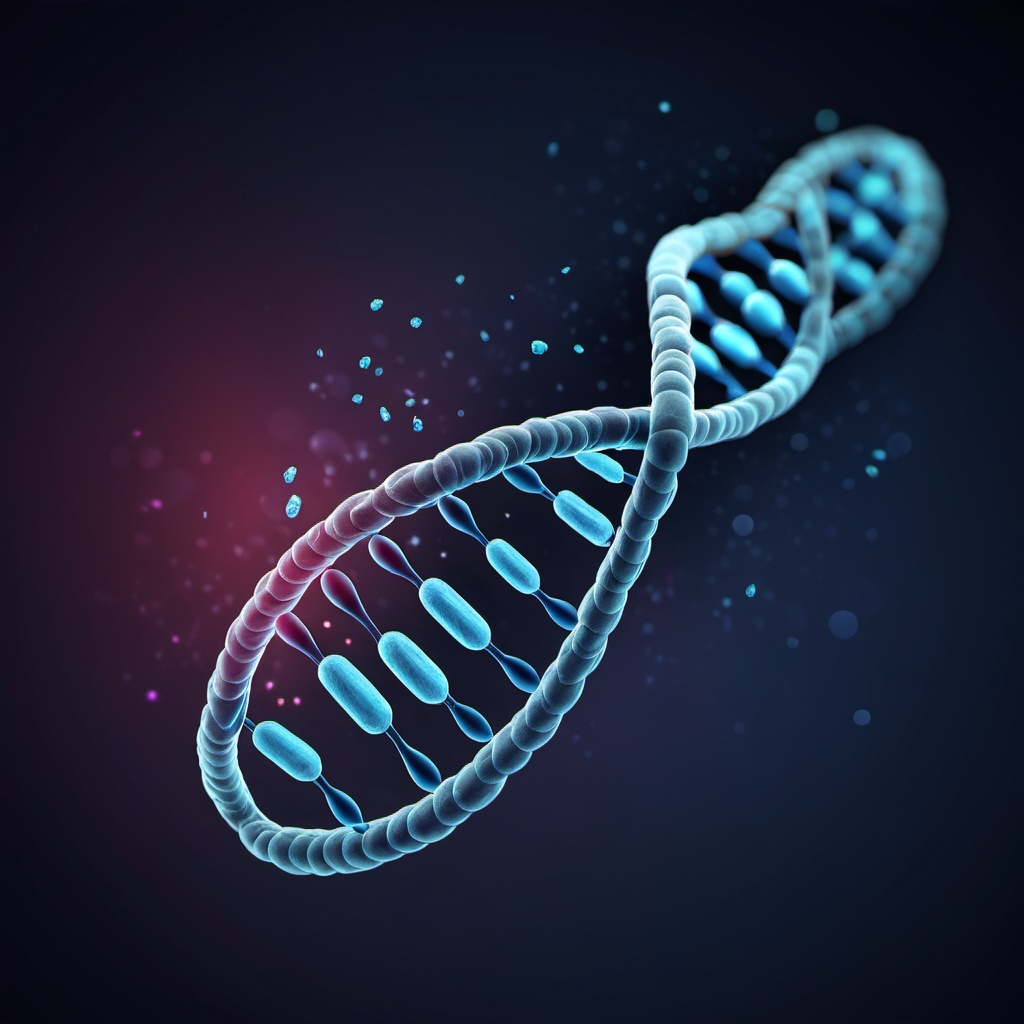
The AI Advantage: Revolutionizing the Drug Discovery Playbook
Introduction
The drug discovery and development process is a complex, time-consuming, and an expensive endeavor that faces numerous challenges in bringing new therapeutics to market. Traditionally, it takes 10-15 years and costs approximately $2.3 billion to develop a single new drug. This lengthy timeline and enormous financial investment are due to the intricate nature of identifying promising drug candidates, conducting extensive preclinical and clinical trials, and navigating regulatory hurdles.
The current drug development process can be broadly divided into four main phases:
- Discovery and Target Identification
- Preclinical studies
- Clinical development
- FDA Market approval
Each stage presents its own set of challenges, from target identification and validation to safety assessment and regulatory compliance. One of the most significant hurdles is the high failure rate of drug candidates, with fewer than 14% of compounds entering phase 1 clinical trials ultimately gaining FDA approval.
Drug discovery and development face significant challenges that hinder the creation of new therapeutics:
- High Costs: Developing a single drug typically costs $2.5 billion and takes 10-15 years, putting immense pressure on pharmaceutical companies.
- Complex Protocols: Clinical trial designs have become increasingly intricate, leading to longer durations and higher costs.
- Patient Recruitment: Identifying and retaining suitable participants for clinical trials is often difficult, especially for rare diseases.
- Limited Disease Understanding: Incomplete knowledge of disease mechanisms complicates the identification of effective drug targets.
- Ineffective Modeling: Traditional disease models, including animal studies, often fail to accurately predict human responses to drugs.
- Regulatory Hurdles: Navigating complex and evolving regulatory requirements across different regions poses significant challenges.
- Data Management: The increasing volume and complexity of biomedical data present difficulties in standardization, integration, and analysis.
To address these issues, the pharmaceutical industry is increasingly turning to innovative technologies like artificial intelligence and machine learning, aiming to improve efficiency and success rates in drug discovery and development.
The AI Opportunity
The landscape of drug development has been fundamentally transformed by the convergence of key technological innovations enabling AI applications in recent years. This transformation stems from major advances in computing infrastructure and specialized AI hardware, alongside an unprecedented expansion in high-quality biological data availability through reduced genomic sequencing costs and comprehensive protein structure databases.
Algorithmic breakthroughs in transformer architectures, graph neural networks, and generative models have revolutionized the analysis of molecular structures, while improvements in laboratory automation and data integration frameworks have streamlined the experimental process. The emergence of specialized chemical AI libraries and multi-modal models has created a synergistic ecosystem where advances in one domain accelerate progress in others, establishing a positive feedback loop between tool development, data generation, and model sophistication.
Advances in AI Technology
- Deep Learning and Neural Networks: The development and refinement of deep learning techniques and neural networks have dramatically improved AI's ability to process complex biological data. These models can now handle vast amounts of data, leading to more accurate predictions in drug discovery.
- Generative AI Models: The introduction of generative AI, which uses deep neural networks to create new data based on learned patterns, has transformed how new drug candidates are identified and optimized. These models can simulate molecular interactions and predict the properties of potential drugs more efficiently than traditional methods.
- Multimodal AI: Recent advancements in multimodal AI allow for the integration of diverse data types, such as genomic, phenotypic, and chemical data, which is crucial for understanding complex biological systems and accelerating drug discovery.
Increased Data Availability
The availability of large datasets from genomic studies, clinical trials, and public repositories has provided the raw material necessary for training sophisticated AI models. This abundance of data enables AI systems to uncover patterns that were previously undetectable.
Computational Power
The rise of powerful computing resources, including GPUs and cloud computing, has made it possible to train large AI models more quickly and cost-effectively. This computational power is essential for processing the complex datasets involved in drug discovery.
Industry Adoption
Pharmaceutical companies are increasingly investing in AI technologies to streamline their research and development processes. The successful application of AI in other industries has demonstrated its potential, encouraging its adoption in drug discovery.
Despite persistent challenges in data quality consistency and model interpretability, this technological confluence has accelerated drug discovery processes, potentially reducing development timelines and costs while improving success rates through more accurate prediction of drug properties and efficacy. The continued evolution of these technologies promises to further revolutionize pharmaceutical development, marking a significant shift in how new therapeutics are discovered and brought to market.
What we’re looking for: The Bespoke Data Advantage
In the rapidly evolving landscape of pharmaceutical development, bespoke datasets have emerged as a critical differentiator for startups leveraging artificial intelligence for drug discovery. While public databases provide foundational knowledge, the development and cultivation of proprietary datasets offers unique competitive advantages that are essential for success in this highly specialized field.
Key Value Drivers of Bespoke Datasets:
- Capture specific biological mechanisms underrepresented in public databases
- Maintain higher quality standards and consistency through controlled conditions
- Enable training of more accurate AI models for specific therapeutic areas
- Create barriers to entry for competitors
- Combine multiple data modalities for comprehensive biological understanding
- Allow for iterative improvement through active learning approaches
The competitive advantage extends beyond mere data possession. Companies that generate their own experimental data can create a virtuous cycle where model predictions guide new experiments, which in turn generate more valuable data. This process builds significant barriers to entry, as competitors would need to invest considerable time and resources to replicate similar datasets.
The strategic importance of bespoke datasets will likely increase as AI technologies become more sophisticated and competition intensifies. Success factors for startups will include:
- Ability to generate high-quality, relevant data efficiently
- Sophisticated data management and analysis capabilities
- Clear alignment between data strategy and therapeutic focus
- Strong validation processes and quality control measures
- Effective integration of multiple data types
- Robust infrastructure for data security and scaling
The success of drug discovery startups in the coming years will increasingly depend on their ability to generate, maintain, and effectively utilize proprietary datasets. We believe those companies that invest in building robust, high-quality datasets tailored to their specific therapeutic areas will be best positioned to capitalize on the transformative potential of AI in drug development.
What we’re excited about: The Rise of Multiomics
The rise of multi-omics in drug discovery marks a significant shift in how pharmaceutical research is conducted, offering a comprehensive systems-level perspective of biological processes. By integrating diverse data types such as genomics, transcriptomics, proteomics, and metabolomics, multi-omics approaches provide unprecedented insights into complex disease mechanisms and potential therapeutic targets. This holistic view is transforming the traditional "one gene, one disease" paradigm into a more dynamic understanding of human biology, paving the way for more effective and precisely targeted therapies.
Background and Significance: Traditional drug discovery approaches have relied heavily on single-pathway analyses, often leading to oversimplified understanding of disease mechanisms and suboptimal therapeutic outcomes. The emergence of multi-omics technologies addresses these limitations by providing a comprehensive view of biological systems across multiple molecular layers. This holistic approach has become increasingly critical as our understanding of disease complexity and system-wide effects has grown.
Current State of the Field: The power of multi-omics lies in its ability to simultaneously analyze multiple molecular layers, enabling researchers to track disease progression, identify novel drug targets and biomarkers, understand drug resistance mechanisms, and stratify patient populations for targeted therapies. Recent advances in data integration methods and computational capabilities have made it possible to meaningfully interpret the vast amounts of data generated through these approaches.
Key Milestones in the Industry:
- First AI-Designed Drug in Clinical Trials (2020): Exscientia announced the first AI-designed drug molecule to enter human clinical trials, marking a significant breakthrough in the field.
- AlphaFold's Protein Structure Prediction (2021): DeepMind's AlphaFold predicted the structures of 330,000 proteins, including all 20,000 in the human genome. The database has since expanded to cover over 200 million proteins.
- AI-Discovered Novel Target and Molecule (2022): Insilico Medicine reported the start of Phase I clinical trials for the first AI-discovered molecule based on an AI-discovered novel target, achieved in a fraction of the time and cost of traditional methods.
- De Novo Antibody Creation (2023): AbSci became the first entity to create and validate de novo antibodies in silico using generative AI.
- FDA Orphan Drug Designation (2023): The FDA granted its first Orphan Drug Designation to a drug discovered and designed using AI, with Insilico Medicine planning to begin a global Phase II trial.
- High Success Rates in Clinical Trials (2024): A study revealed that AI-derived molecules have a success rate of 80–90% in clinical trials, significantly higher than historical averages.
The rise of multi-omics in drug discovery represents a fundamental shift in therapeutic development, moving from reductionist approaches to a more comprehensive understanding of biological systems. This transition promises to improve success rates in drug development while potentially reducing costs and development timelines. The continued evolution of multi-omics technologies, coupled with advances in computational capabilities, suggests that this approach will become increasingly central to drug discovery efforts. As these technologies continue to mature and integrate with other advancing fields, they will likely become an indispensable component of modern drug discovery, enabling more precise, effective, and personalized therapeutic development.
Conclusion
The field of AI-driven drug discovery is poised for continued rapid evolution. As computational capabilities advance and datasets expand, we anticipate even more sophisticated applications of AI in target identification, lead optimization, and clinical trial design. The success of early AI-discovered drug candidates in clinical trials suggests we are at the beginning of a transformative era in pharmaceutical development.
For founders building in this space, the opportunity to contribute to this transformative era in drug discovery is both exciting and impactful. If you are a founder leveraging AI technologies to innovate in drug discovery, we invite you to reach out.
If you’re a founder building in any of these areas I’d love to hear from you! Please reach out to Nia/Daphne at nia@montageventures.com / daphne@montageventures.com